The Computational Engineering and Analysis research group at Turku University of Applied Sciences used the data from the machine learning model to build an ideal pace distribution strategy that the right athlete can use in their training. Eero Immonen, the leader of the research group, sees that the optimisation method can be applied to the design of battery-powered machines.
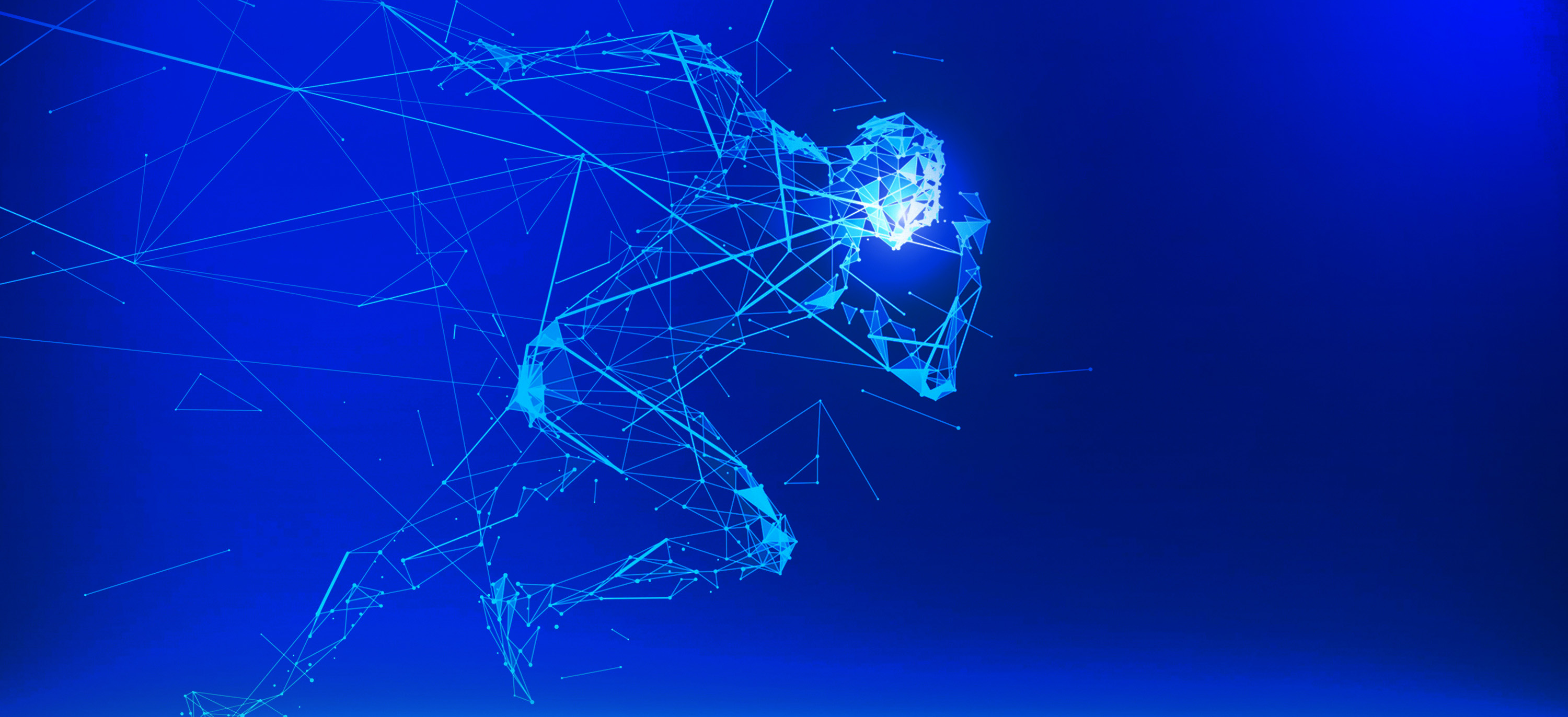
Published:
Edited:
Text: by Siiri Welling
How to optimize the energy use of equipment or even people to improve results?
Eero Immonen, senior lecturer and researcher at Turku University of Applied Sciences, now has a clear answer to this question: mathematics. More precisely, by using a machine learning model and calculations that are more than 50 years old.
“It actually all started from an earlier project at Turku University of Applied Sciences, e3Power . We were developing battery models, but the development was kind of left unfinished. Now we were looking at an optimization solution for existing equipment that would tell us how to use the battery system to get the most out of it.”
The recent report by Immonen’s research group comes at a very timely moment. World transport has long been electrifying at breakneck speed, and the next step will be the electrification of machinery. Mining company Sandvik, for example, has announced that it will start manufacturing battery-powered mining machines. While the research team’s report focuses on the right human locomotion and finding the right pacing strategy, the problem is the same as with battery-powered machines: how to perform a repetitive or well-defined task in the most energy-efficient way? In the case of machine tools, battery operation could be optimized, for example, to get the machine up from the mine to the surface as quickly as possible.
“For both machine tools and humans, movement is limited by the laws of physics and the limited energy system of the person moving. The optimum solution is well known, so the optimization method was studied to ensure that it works,” says Immonen.
Immonen and his research team used the laws of physics to think about optimisation. The use of force enables movement, but there is only a limited amount of force that can be produced. The use of power and energy should therefore be designed intelligently to meet the objectives of mobility. For example, consider the acceleration of a machine: it is generally known that rapid acceleration consumes energy, but for battery-powered machines it is advisable to consider whether high energy consumption is reasonable. Until the Immonen group’s research, calculations using machine learning had not been carried out in such a way that a computational solution would tell us what the most optimal way of using energy would be.
In effect, the computer learned to run world records using a new speed distribution strategy.
Human running speed has already been studied
Immonen’s research team approached the problem using the research data of the American mathematician Joseph Keller. In 1973, Keller used mathematical models to study the distribution of speeds in world record races. Keller collected data for 400 metres, 1 500 metres and 10 000 metres. Over the years, others have also developed mathematical methods to improve runners’ performance, but without using machine learning.
“We used reinforcement learning, a type of machine learning based on reinforcement. We fed into a computer the data Keller collected in the 1970s, and through tens of thousands of virtual running trials, the computer calculated the optimal way for us to run in order to set a new world record. In effect, the computer learned how to run world records using a new speed distribution strategy,” explains Immonen.
The research was very successful. Especially for longer distances, over 1 500 meters, the speed profiles created by Immonen’s research team are almost identical to the solutions proposed by Keller. Sajad Shahsavari, a special researcher at Turku University of Applied Sciences, carried out much of the successful research.
The results of Immonen’s research group could be applied to the training programme of today’s athletes, although this would first require collecting a new type of data from the athlete. Immonen points out that each athlete is a unique entity, and optimization of this kind should take into account the athlete’s own metabolism. Optimization methods are particularly suitable for tasks with a clear goal and where it is necessary to limit the energy source.
“The computational method has a wide range of applications. The fact that we were able to reproduce Keller’s results gives important credibility to work machine applications where model solutions are not available,” concludes Immonen.
The article was published on 24.11.2022 on the previous turkuamk.fi website.
Get to know our Research
Read next
-
Press Release
Recent study: multi-storey office noise problems transnational but solvable
Globally, the biggest indoor environmental problem in open offices is noise from colleagues. However, this problem can be solved by investing in room acoustics and soundproof office cubicles.…
-
Press Release
Turku University of Applied Sciences receives EUR 2.4 million in funding to develop a sustainable shipbuilding ecosystem
Turku University of Applied Sciences’ project Sustainable Material Management Maturity Model (S4M) has received Business Finland’s co-innovation research funding as part of Meyer Turku’s NecOLEAP locomotive project programme.…
-
Case
Sustainable mobility in urban areas – Turku University of Applied Sciences developed fast charging stations for electric bikes
Fast-charging stations for electric bicycles have been introduced in Turku. The new energy research group at Turku University of Applied Sciences implemented the stations as part of a…