PHASE IV AI
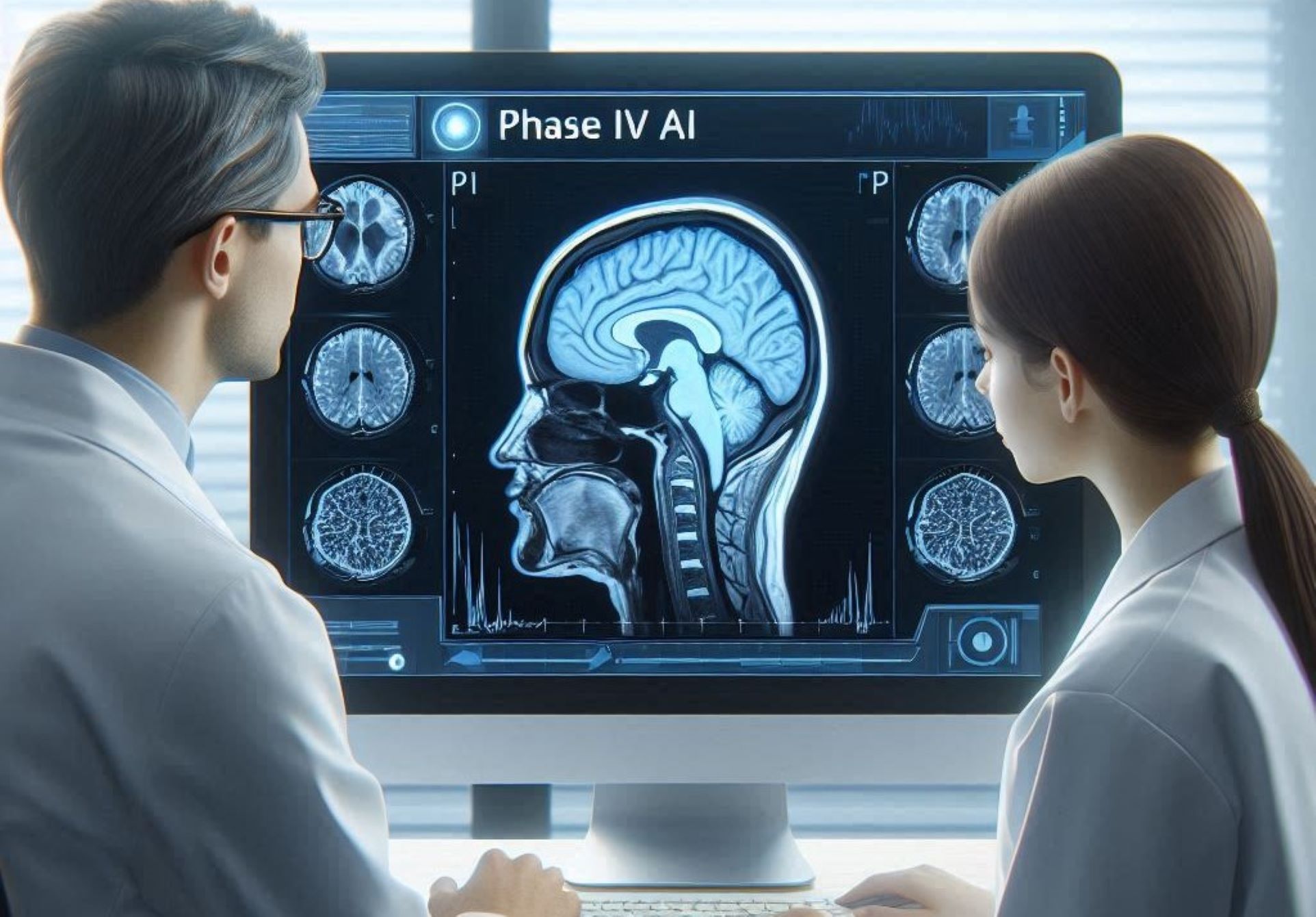
Project duration
Source of funding
Horizon Europe
Total funding
7 000 000 €
Data driven tools (esp. AI) usually need a large amount of data for achieving relevance and accuracy. Therefore, development of such tools for healthcare solutions requires broad applicability of validated data sets of appropriate size depending on large populations. However, the application of data for healthcare today is limited due to the sensitive nature of health data and potential high privacy impact.
Data-driven approaches may not work for diseases with a small local population. In this case, de-identification often is not strong enough or today’s de-identifcation strategies do not lead to the required accuracy in detection of prediction. Thus , such kind of diseases cannot be tackled by AI based technology due to limitations of existing anonymisation or synthetization techniques. Synthetic data and federated learning assist in this because they facilitate data sharing without compromising data security.
The usefulness of synthetic data is not obvious. Usefulness usually is unclear before tackling the real problem and validating the synthetic data in comparison with underlying real world data in specific cases. The criteria, how synthetic data shall be generated in a generally useful way currently are subject to research. Type of data, data generation mechanism need to be analysed for a more generalized approach. Moreover, quality evaluation and validation tools are lacking as well a quality metrics measuring utility & privacy criteria.
Objectives:
- Improved Technologies for (federated) anonymisation, synthetization of health data with strong de-identification properties
- Enable AI developers access to larger pools of data for federated learning by easy to use and configurable data services
- Establish a Data Market – facilitating data sharing and monetization incl. incentives based providing data to the services
- Integrate the data market and the data service ecosystem as a X-European health data hub in the European Health Data Space.
We at Turku UAS will investigate local and global weight aggregation models tailored with differential privacy techniques to develop a scalable and multi-layer secure Federated Learning systems for privacy preservation in health data setting. We will also investigate methods to assess generated synthetic image data from clinical and privacy points of view. We will consider statistical and mathematical techniques to evaluate synthetic data generated by AI models privacy guarantees for the FL and DP models.
Picture: Jussi Salmi / AI Copilot, 09/2024
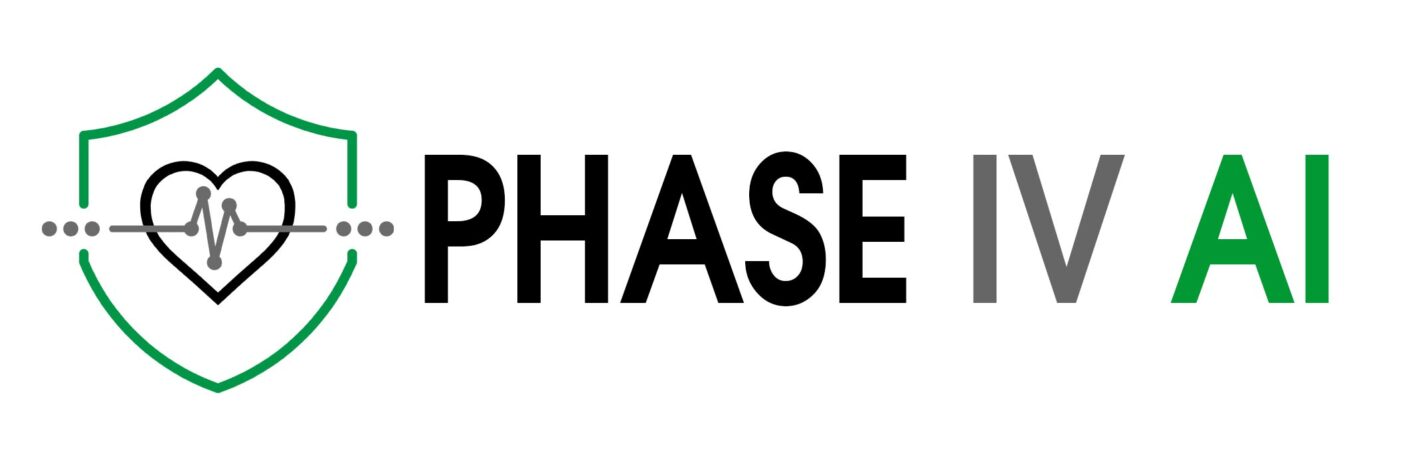
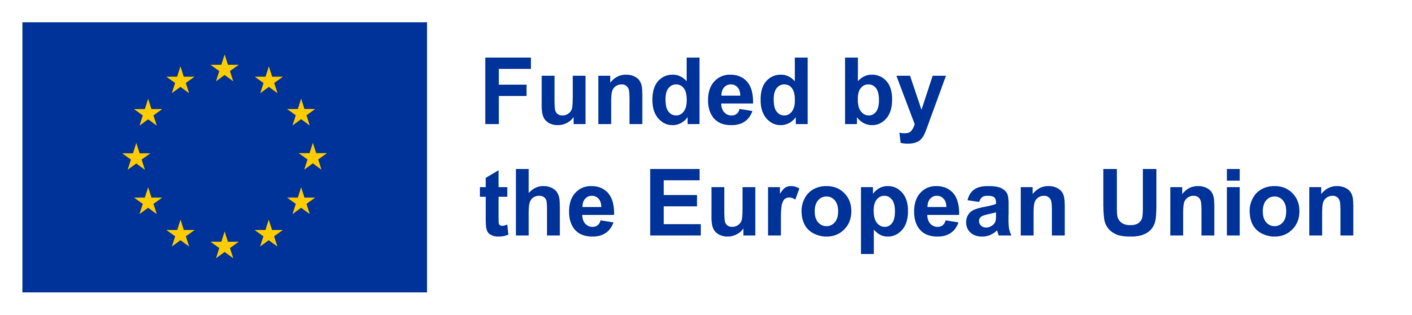
Contact Us
Partners
- Ainigma Technologies, Spain
- Centre Hospitalier Universitaire Vaudois, Switzerland
- Engineering – Ingegneria Informatica SPA, Italy
- Fujitsu Technology Solutions SA N/V, Belgium
- Fujitsu Technology Solutions SA, Luxemburg
- Fundacio Eurecat, Spain
- Fundacio Hospital Universitari Vall D’Hebron – Institut de Recerca, Spain
- INESC TEC – Instituto de Engenhariade Sistemas e Computadores, Tecnologia e Ciensia, Portugal
- Inpher SARL, Switzerland
- Katholieke Universiteit LEUVEN, Belgium
- LeanXcale SL, Spain
- Nottingham University Hospitals NHS Trust, UK
- Resilience Guard GMBH, Switzerland
- Sabanci Universitesi, Turkey
- Turku University Hospital TYKS, Finland
- Universitat Wien, Austria
- University Nottingham Trent University, UK
- University of Turku, Finland (Coordinator)
- VTT Technical Research Centre of Finland
Get to know the research group
-
Research groups
Health Technology
See all our projects
We carry out nearly 200 RDI projects annually together with working life and our international partner networks.